This era is called to be ✅ Artificial intelligence (AI) and Internet of Things (IoT), it is operated by robots and they are replaced the human. This article we mention the Artificial Intelligence for CCTV Cameras, Video Surveillance, it applies for this industry.
- What is Artificial Intelligence ?
-
Artificial intelligence technology for CCTV Cameras
-
Artificial intelligence solutions for CCTV Cameras Companies
Read more: How to Detect Hidden Cameras ? [EASY with 6 SIMPLE Steps]
There has never been a better time to protect your property with CCTV; modern innovations have allowed security camera companies to create high-definition, fully manoeuvrable, internet-connecting cameras that provide their operators with an even wider range of flexibility than ever before. So where next for the CCTV camera industry?
Regardless of the industry in mind, automation seems to be the next step in their evolution – and CCTV is no different. Machine learning techniques are being tested in the hope that they’ll provide CCTV cameras with the ability to spot ‘troubling behaviour’ without the need for a human operator.
What is Artificial Intelligence ?
Artificial intelligence (AI) is an area of computer science that emphasizes the creation of intelligent machines that work and react like humans. Some of the activities computers with artificial intelligence are designed for include:
- Speech recognition
- Learning
- Planning
- Problem solving
Techopedia explains Artificial Intelligence (AI)
Artificial intelligence is a branch of computer science that aims to create intelligent machines. It has become an essential part of the technology industry.
Research associated with artificial intelligence is highly technical and specialized. The core problems of artificial intelligence include programming computers for certain traits such as:
- Knowledge
- Reasoning
- Problem solving
- Perception
- Learning
- Planning
- Ability to manipulate and move objects
Knowledge engineering is a core part of AI research. Machines can often act and react like humans only if they have abundant information relating to the world.
Artificial intelligence must have access to objects, categories, properties and relations between all of them to implement knowledge engineering. Initiating common sense, reasoning and problem-solving power in machines is a difficult and tedious task.
- Machine learning is also a core part of AI. Learning without any kind of supervision requires an ability to identify patterns in streams of inputs, whereas learning with adequate supervision involves classification and numerical regressions. Classification determines the category an object belongs to and regression deals with obtaining a set of numerical input or output examples, thereby discovering functions enabling the generation of suitable outputs from respective inputs. Mathematical analysis of machine learning algorithms and their performance is a well-defined branch of theoretical computer science often referred to as computational learning theory.
- Machine perception deals with the capability to use sensory inputs to deduce the different aspects of the world, while computer vision is the power to analyze visual inputs with a few sub-problems such as facial, object and gesture recognition.
- Robotics is also a major field related to AI. Robots require intelligence to handle tasks such as object manipulation and navigation, along with sub-problems of localization, motion planning and mapping.
Reference: How To Install CCTV Cameras ? [ Easy With 7 Simple Steps]
Artificial intelligence Technology for CCTV Cameras / Video surveillance
- Artificial intelligence for CCTV Cameras or video surveillance utilizes computer software programs that analyze the images from video surveillance cameras in order to recognize humans, vehicles or objects. Security contractors program the software to define restricted areas within the camera’s view (such as a fenced off area, a parking lot but not the sidewalk or public street outside the lot) and program for times of day (such as after the close of business) for the property being protected by the camera surveillance. The artificial intelligence (“A.I.”) sends an alert if it detects a trespasser breaking the “rule” set that no person is allowed in that area during that time of day.
- The A.I. program functions by using machine vision. Machine vision is a series of algorithms, or mathematical procedures, which work like a flow-chart or series of questions to compare the object seen with hundreds of thousands of stored reference images of humans in different postures, angles, positions and movements. The A.I. asks itself if the observed object moves like the reference images, whether it is approximately the same size height relative to width, if it has the characteristic two arms and two legs, if it moves with similar speed, and if it is vertical instead of horizontal. Many other questions are possible, such as the degree to which the object is reflective, the degree to which it is steady or vibrating, and the smoothness with which it moves. Combining all of the values from the various questions, an overall ranking is derived which gives the A.I. the probability that the object is or is not a human. If the value exceeds a limit that is set, then the alert is sent. It is characteristic of such programs that they are self-learning to a degree, learning, for example that humans or vehicles appear bigger in certain portions of the monitored image – those areas near the camera – than in other portions, those being the areas farthest from the camera.
- In addition to the simple rule restricting humans or vehicles from certain areas at certain times of day, more complex rules can be set. The user of the system may wish to know if vehicles drive in one direction but not the other. Users may wish to know that there are more than a certain preset number of people within a particular area. The A.I. is capable of maintaining surveillance of hundreds of cameras simultaneously. Its ability to spot a trespasser in the distance or in rain or glare is superior to humans’ ability to do so.
- This type of A.I. for security is known as “rule-based” because a human programmer must set rules for all of the things for which the user wishes to be alerted. This is the most prevalent form of A.I. for security. Many video surveillance camera systems today include this type of A.I. capability. The hard-drive that houses the program can either be located in the cameras themselves or can be in a separate device that receives the input from the cameras.
- A newer, non-rule based form of A.I. for security called “behavioral analytics” has been developed. This software is fully self-learning with no initial programming input by the user or security contractor. In this type of analytics, the A.I. learns what is normal behavior for people, vehicles, machines, and the environment based on its own observation of patterns of various characteristics such as size, speed, reflectivity, color, grouping, vertical or horizontal orientation and so forth.
- The A.I. normalizes the visual data, meaning that it classifies and tags the objects and patterns it observes, building up continuously refined definitions of what is normal or average behavior for the various observed objects. After several weeks of learning in this fashion it can recognize when things break the pattern. When it observes such anomalies it sends an alert. For example, it is normal for cars to drive in the street. A car seen driving up onto a sidewalk would be an anomaly. If a fenced yard is normally empty at night, then a person entering that area would be an anomaly.
Read more: [10 secret methods] to hack security cameras from hackers
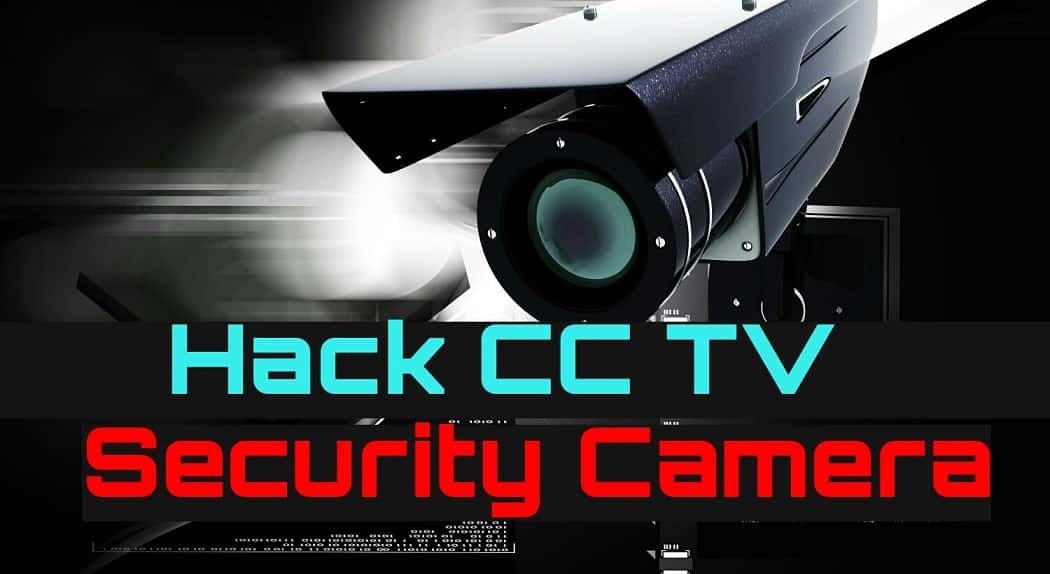
The advances in CCTV technology are due to two factors:
Advanced object motion detection:
- With previous technology the cameras were only able to do limited object detection, such as distinguishing between a person walking and a car driving. “Deep learning algorithms now enable video monitoring systems to figure out specific details about what cameras are seeing. This includes more granular information such as if the person in the video is a woman or a man, and what color his or her clothes are,” he writes.
- The next evolution reduced false alerts to a degree but at the cost of complicated and time-consuming manual calibration. Here, changes of a target such as a person or vehicle relative to a fixed background are detected. Where the background changes seasonally or due to other changes, the reliability deteriorates over time. The economics of responding to too many false alerts again proved to be an obstacle and this solution was insufficient.
In-depth behavioral analysis:
- Previously, human operators had to view video recordings to try to figure out emotions or reactions from facial expressions caught on camera. “Analytics technology can now teach cameras how to read micro-expressions, helping marketers and behavioral analysts alike understand how their customers feel during their experience. Smart cameras, for example, can determine if a shopper is excited or confused when presented with different retail advertisements or product displays.”
- Machine learning of visual recognition relates to patterns and their classification. True video analytics can distinguish the human form, vehicles and boats or selected objects from the general movement of all other objects and visual static or changes in pixels on the monitor. It does this by recognizing patterns. When the object of interest, for example a human, violates a preset rule, for example that the number of people shall not exceed zero in a pre-defined area during a defined time interval, then an alert is sent. A red rectangle or so-called “bounding box” will typically automatically follow the detected intruder, and a short video clip of this is sent as the alert.
Behavioral analytics
Active environments
- While rule-based video analytics worked economically and reliably for many security applications there are many situations in which it cannot work. For an indoor or outdoor area where no one belongs during certain times of day, for example overnight, or for areas where no one belongs at any time such as a cell tower, traditional rule-based analytics are perfectly appropriate.
- In the example of a cell tower the rare time that a service technician may need to access the area would simply require calling in with a pass-code to put the monitoring response “on test” or inactivated for the brief time the authorized person was there.
- But there are many security needs in active environments in which hundreds or thousands of people belong all over the place all the time. For example, a college campus, an active factory, a hospital or any active operating facility. It is not possible to set rules that would discriminate between legitimate people and criminals or wrong-doers.
Overcoming the problem of active environments
- Using behavioral analytics, a self-learning, non-rule-based A.I. takes the data from video cameras and continuously classifies objects and events that it sees. For example, a person crossing a street is one classification. A group of people is another classification. A vehicle is one classification, but with continued learning a public bus would be discriminated from a small truck and that from a motorcycle. With increasing sophistication, the system recognizes patterns in human behavior.
- For example, it might observe that individuals pass through a controlled access door one at a time. The door opens, the person presents their proximity card or tag, the person passes through and the door closes. This pattern of activity, observed repeatedly, forms a basis for what is normal in the view of the camera observing that scene.
- Now if an authorized person opens the door but a second “tail-gating” unauthorized person grabs the door before it closes and passes through, that is the sort of anomaly that would create an alert. This type of analysis is much more complex than the rule-based analytics. While the rule-based analytics work mainly to detect intruders into areas where no one is normally present at defined times of day, the behavioral analytics works where people are active to detect things that are out of the ordinary.
- A fire breaking out outdoors would be an unusual event and would cause an alert, as would a rising cloud of smoke. Vehicles driving the wrong way into a one-way driveway would also typify the type of event that has a strong visual signature and would deviate from the repeatedly observed pattern of vehicles driving the correct one-way in the lane. Someone thrown to the ground by an attacker would be an unusual event that would likely cause an alert. This is situation-specific. So if the camera viewed a gymnasium where wrestling was practiced the A.I. would learn it is usual for one human to throw another to the ground, in which case it would not alert on this observation.
What the artificial intelligence ‘understands’
- The A.I. does not know or understand what a human is, or a fire, or a vehicle. It is simply finding characteristics of these things based on their size, shape, color, reflectivity, angle, orientation, motion, and so on. It then finds that the objects it has classified have typical patterns of behavior.
- For example, humans walk on sidewalks and sometimes on streets but they don’t climb up the sides of buildings very often. Vehicles drive on streets but don’t drive on sidewalks. Thus the anomalous behavior of someone scaling a building or a vehicle veering onto a sidewalk would trigger an alert.
Varies from traditional mindset of security systems
- Typical alarm systems are designed to not miss true positives (real crime events) and to have as low of a false alarm rate as possible. In that regard, burglar alarms miss very few true positives but have a very high false alarm rate even in the controlled indoor environment.
- Motion detecting cameras miss some true positives but are plagued with overwhelming false alarms in an outdoor environment. Rule-based analytics reliably detect most true positives and have a low rate of false positives but cannot perform in active environments, only in empty ones. Also they are limited to the simple discrimination of whether an intruder is present or not.
- Something as complex or subtle as a fight breaking out or an employee breaking a safety procedure is not possible for a rule based analytics to detect or discriminate. With behavioral analytics, it is. Places where people are moving and working do not present a problem. However, the A.I. may spot many things that appear anomalous but are innocent in nature.
- For example, if students at a campus walk on a plaza, that will be learned as normal. If a couple of students decided to carry a large sheet outdoors flapping in the wind, that might indeed trigger an alert. The monitoring officer would be alerted to look at his or her monitor and would see that the event is not a threat and would then ignore it. The degree of deviation from norm that triggers an alert can be set so that only the most abnormal things are reported.
- However, this still constitutes a new way of human and A.I. interaction not typified by the traditional alarm industry mindset. This is because there will be many false alarms that may nevertheless be valuable to send to a human officer who can quickly look and determine if the scene requires a response. In this sense, it is a “tap on the shoulder” from the A.I. to have the human look at something.
Limitations of behavioral analytics
- Because so many complex things are being processed continuously, the software samples down to the very low resolution of only 1 CIF to conserve computational demand. The 1 CIF resolution means that an object the size of a human will not be detected if the camera utilized is wide angle and the human is more than sixty to eighty feet distant depending on conditions. Larger objects like vehicles or smoke would be detectable at greater distances.
Quantification of situational awareness
- The utility of artificial intelligence for security does not exist in a vacuum, and its development was not driven by purely academic or scientific study. Rather, it is addressed to real world needs, and hence, economic forces. Its use for non-security applications such as operational efficiency, shopper heat-mapping of display areas (meaning how many people are in a certain area in a retail space), and attendance at classes are developing uses. Humans are not as well qualified as A.I. to compile and recognize patterns consisting of very large data sets requiring simultaneous calculations in multiple remote viewed locations.
- There is nothing natively human about such awareness. Such multi-tasking has been shown to defocus human attention and performance. A.I.s have the ability to handle such data. For the purposes of security interacting with video cameras they functionally have better visual acuity than humans or the machine approximation to it. For judging subtleties of behaviors or intentions of subjects or degrees of threat, humans remain far superior at the present state of the technology. So the A.I. in security functions to broadly scan beyond human capability and to vet the data to a first level of sorting of relevance and to alert the human officer who then takes over the function of assessment and response.
- Security in the practical world is economically determined so that the expenditure of preventative security will never typically exceed the perceived cost of the risk to be avoided. Studies have shown that companies typically only spend about one twenty-fifth the amount on security that their actual losses cost them. What by pure economic theory should be an equivalence or homeostasis, thus falls vastly short of it.
- One theory that explains this is cognitive dissonance, or the ease with which unpleasant things like risk can be shunted from the conscious mind. Nevertheless, security is a major expenditure, and comparison of the costs of different means of security is always foremost amongst security professionals.
- Another reason that future security threats or losses are under-assessed is that often only the direct cost of a potential loss is considered instead of the spectrum of consequential losses that are concomitantly experienced. For example, the vandalism-destruction of a custom production machine in a factory or of a refrigerated tractor trailer would result in a long replacement time during which customers could not be served, resulting in loss of their business. A violent crime will have extensive public relations damage for an employer, beyond the direct liability for failing to protect the employee.
- Behavioral analytics uniquely functions beyond simple security and, due to its ability to observe breaches in standard patterns of protocols, it can effectively find unsafe acts of employees that may result in workers comp or public liability incidents. Here too, the assessment of future incidents’ costs falls short of the reality.
- A study by Liberty Mutual Insurance Company showed that the cost to employers is about six times the direct insured cost, since uninsured costs of consequential damages include temporary replacement workers, hiring costs for replacements, training costs, managers’ time in reports or court, adverse morale on other workers, and effect on customer and public relations. The potential of A.I. in the form of behavioral analytics to proactively intercept and prevent such incidents is significant.
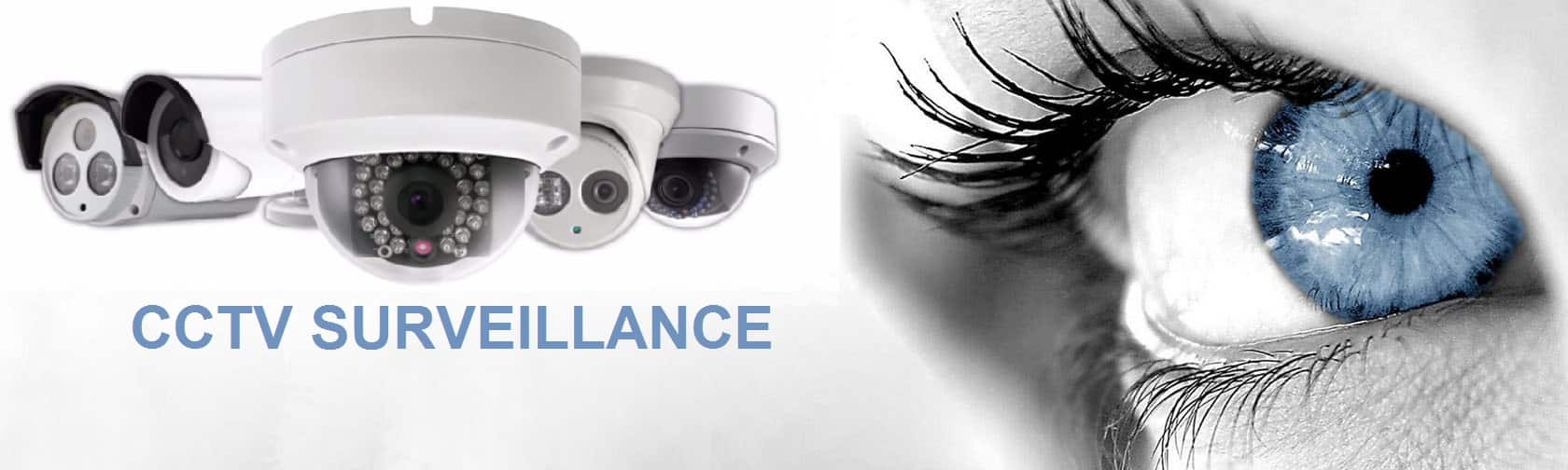
AI CCTV – The Next Big Thing For Security Camera Companies?
- The Japanese telecom giant, NTT East and security camera provider, Earth Eyes Corp, have teamed up to create and test a new camera that uses artificial intelligence to operate. Entitled the ‘AI Guardman’, the camera takes advantage of open source technology (developed by Carnegie Mellon University) to scan live video streams and estimate the body positions it can see.
- In their article on the news, The Verge has compared it to a Microsoft Kinect camera, which gives users the ability to control and interact with their computer without the need for a controller. Once the AI Guardman matches a body position with predefined data it holds regarding ‘suspicious behaviour’, it will alert the operator via an app.
- Having been in development for a number of years, it’s only recently that NTT East and Earth Eyes have been able to test their AI wireless security camera. Initial tests seem to have been positive – The Verge report that the AI Guardman apparently reduced shoplifting incidents by around 40 per cent in the stores it was tested in. However, like the article states – these results should be taken with a pinch of salt (or probably a fistful); it’s unknown what type of security camera the tested stores already had (if any), to compare to the AI camera.
- However, the theory behind the idea is a solid one – AI is increasingly growing in influence in our world; you just have to look how our mobile phones use voice searches or how websites present tailored adverts to us to understand the influence machine learning already has. Not having to worry about the security of their store, AI will eventually give business owners and staff alike the ability to better define their roles, opening themselves up to a new range of possibilities.
- Realistically, the idea of an AI security camera that is able to perform effectively is something that will require many years of further development and many more beyond that before it can become affordable for everyone.
Artificial intelligence solutions for CCTV Cameras Companies
Artificial Intelligence for CCTV Cameras – Hikvision AI Cloud
References:
- https://en.wikipedia.org/wiki/Artificial_intelligence_for_video_surveillance
- https://www.techopedia.com/definition/190/artificial-intelligence-ai
- https://www.hikvision.com
Read more:
- Top 10 CCTV Camera Brands Global Ranking by Asmag